Semi-supervised Log-based Anomaly Detection via Probabilistic Label Estimation
Technical Track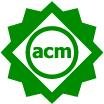
Thu 27 May 2021 02:30 - 02:50 at Blended Sessions Room 3 - 2.3.3. Software Log Analysis
With the growth of software systems, logs have become an important data to aid system maintenance. Log-based anomaly detection is one of the most important methods for such purpose, which aims to automatically detect system anomalies via log analysis. However, existing log-based anomaly detection approaches still suffer from practical issues due to either depending on a large amount of manually labeled training data (supervised approaches) or unsatisfactory performance without learning the knowledge on historical anomalies (unsupervised and semi-supervised approaches). In this paper, we propose a novel practical log-based anomaly detection approach, PLELog, which is semi-supervised to get rid of time-consuming manual labeling and incorporates the knowledge on historical anomalies via probabilistic label estimation to bring supervised approaches’ superiority into play. In addition, PLELog is able to stay immune to unstable log data via semantic embedding and detect anomalies efficiently and effectively by designing an attention-based GRU neural network. We evaluated PLELog on two most widely-used public datasets, and the results demonstrate the effectiveness of PLELog, significantly outperforming the compared approaches with an average of 181.6% improvement in terms of F1-score. In particular, PLELog has been applied to two real-world systems from our university and a large corporation, further demonstrating its practicability.
Wed 26 MayDisplayed time zone: Amsterdam, Berlin, Bern, Rome, Stockholm, Vienna change
14:30 - 15:25 | 2.3.3. Software Log AnalysisNIER - New Ideas and Emerging Results / Technical Track at Blended Sessions Room 3 +12h Chair(s): Silverio Martínez-Fernández UPC-BarcelonaTech | ||
14:30 20mPaper | Semi-supervised Log-based Anomaly Detection via Probabilistic Label Estimation Technical Track Lin Yang College of Intelligence and Computing, Tianjin University, Junjie Chen College of Intelligence and Computing, Tianjin University, Zan Wang College of Intelligence and Computing, Tianjin University, Weijing Wang College of Intelligence and Computing, Tianjin University, Jiajun Jiang College of Intelligence and Computing, Tianjin University, Xuyuan Dong Information and Network Center,Tianjin University, Wenbin Zhang Information and Network Center,Tianjin University Pre-print Media Attached | ||
14:50 20mPaper | DeepLV: Suggesting Log Levels Using Ordinal Based Neural NetworksTechnical Track Technical Track Zhenhao Li Concordia University, Heng Li Polytechnique Montréal, Tse-Hsun (Peter) Chen Concordia University, Weiyi Shang Concordia University Pre-print Media Attached | ||
15:10 15mShort-paper | On Automatic Parsing of Log RecordsNIER NIER - New Ideas and Emerging Results Pre-print Media Attached |
Thu 27 MayDisplayed time zone: Amsterdam, Berlin, Bern, Rome, Stockholm, Vienna change
02:30 - 03:25 | 2.3.3. Software Log AnalysisTechnical Track / NIER - New Ideas and Emerging Results at Blended Sessions Room 3 | ||
02:30 20mPaper | Semi-supervised Log-based Anomaly Detection via Probabilistic Label Estimation Technical Track Lin Yang College of Intelligence and Computing, Tianjin University, Junjie Chen College of Intelligence and Computing, Tianjin University, Zan Wang College of Intelligence and Computing, Tianjin University, Weijing Wang College of Intelligence and Computing, Tianjin University, Jiajun Jiang College of Intelligence and Computing, Tianjin University, Xuyuan Dong Information and Network Center,Tianjin University, Wenbin Zhang Information and Network Center,Tianjin University Pre-print Media Attached | ||
02:50 20mPaper | DeepLV: Suggesting Log Levels Using Ordinal Based Neural NetworksTechnical Track Technical Track Zhenhao Li Concordia University, Heng Li Polytechnique Montréal, Tse-Hsun (Peter) Chen Concordia University, Weiyi Shang Concordia University Pre-print Media Attached | ||
03:10 15mShort-paper | On Automatic Parsing of Log RecordsNIER NIER - New Ideas and Emerging Results Pre-print Media Attached |